File Dump Untuk Receiver Tanaka
We present a major update of the HOCOMOCO collection that consists of patterns describing DNA binding specificities for human and mouse transcription factors. In this release, we profited from a nearly doubled volume of published in vivo experiments on transcription factor (TF) binding to expand the repertoire of binding models, replace low-quality models previously based on in vitro data only and cover more than a hundred TFs with previously unknown binding specificities.
This was achieved by systematic motif discovery from more than five thousand ChIP-Seq experiments uniformly processed within the BioUML framework with several ChIP-Seq peak calling tools and aggregated in the GTRD database. HOCOMOCO v11 contains binding models for 453 mouse and 680 human transcription factors and includes 1302 mononucleotide and 576 dinucleotide position weight matrices, which describe primary binding preferences of each transcription factor and reliable alternative binding specificities.
Masukan nomor handphone Anda untuk download. Satellite Receiver Set-up Box TV HDTV UK. Tanaka T-21 HD New Sakura Receiver Parabola. Sw v2 matrix terbaru 65 bisa download disini Gan Download software. Lenovo VIBE P1ma40 HDTV Receiver. LNB HDTV DUAL OUT; RECEIVER. Tool buat upgrade tanaka. Software download firmware matrix burger.
- Jun 5, 2017 - In addition, we evaluated our approaches using receiver operating. Files were converted to FASTQ format using the fastq-dump utility of. Toyooka K, Narisawa T, Mochida K, Tanaka H, Matsuda F, Hirai A, Hirai MY, et al.
- Solusi Kehilangan Channel pada receiver Tanaka Sakura Samurai Jurassic Burger S2 via Load Dump File 14 Oktober 2017 - Kategori Blog. Bagi anda yang memiliki masalah channel kosong maupun sedikit, hal ini dialami umumnya pada receiver yang baru di beli, baru di upgrade atau bahkan baru di factory reset.

An interactive interface and bulk downloads are available on the web: and. In this release, we complement HOCOMOCO by MoLoTool (Motif Location Toolbox, ) that applies HOCOMOCO models for visualization of binding sites in short DNA sequences.
INTRODUCTION Models of transcription factor binding sites (TFBS) are essential tools for computational studies of transcriptional regulation from dissection of particular cis-regulatory regions and genome-wide TFBS predictions to modeling regulatory networks and functional annotation of sequence variants (–). Advanced TFBS models evolve rapidly (–), but the basic position weight matrix model remains a useful baseline for a wide range of applications (,–). The wide availability of experimental data on protein-DNA interaction in vivo allows for systematic construction and comparison of different TFBS models. In the past few years, HOCOMOCO database of transcription factor binding models became one of the major resources for sequence analysis of transcriptional regulation in mammals.
In particular, HOCOMOCO has been a useful data source in a recent DREAM-ENCODE challenge on the prediction of transcription factor binding sites where several top-performing teams used HOCOMOCO models in their solutions. Here we present a major update of the HOCOMOCO collection of human and mouse transcription factor binding models based on systematic motif discovery and cross-validation using more than 14 thousand ChIP-Seq data sets obtained from 5000 experiments for human and mouse transcription factors. Such large-scale analysis allowed for significant expansion and improvement of the non-redundant set of TFBS models for human and mouse transcription factors. The diverse repertoire of experimental data sets systematically brings about alternative binding models for a particular TF. Following the original ideas used in developing of HOCOMOCO, we focus on primary binding patterns that robustly represent binding sites across multiple experiments (HOCOMOCO-11-CORE). At the same time, the alternative models are now systematically provided in the extended collection (HOCOMOCO-11-FULL), that now also contains lower-reliability binding models built from limited experimental data. The total number of available ChIP-Seq data sets more than doubled since the previous release (HOCOMOCO v10).
Each ChIP-Seq data set was processed with four different peak callers ( macs, gem, pics, sissrs) (–), thus increasing the diversity of peak sets used for motif discovery: the total number of peak sets became more than ten fold greater compared to the previous HOCOMOCO version. Furthermore, we systematically assessed the suitability of peak callers for downstream motif discovery by comparing TFBS recognition quality of the TBFS models derived from the respective peaks. Finally, profiting from an outstandingly large number of ChIP-Seq datasets for human and mouse species we performed a cross-species validation of human/mouse binding sites models for orthologous transcription factors. We continue to maintain the set of command-line tools to facilitate practical utilization of the HOCOMOCO models: SPRY-SARUS for motif finding (search for motif occurrences), MACRO-APE for weight matrix comparison and P-value estimation, and PERFECTOS-APE for annotation of regulatory variants. The accompanying tools are web-accessible at including ChIPMunk that has been used for motif discovery.
Finally, in this version of HOCOMOCO, we introduce MoLoTool (Motif Location Toolbox), an interactive web-tool to identify motif occurrences in a set of sequences based on HOCOMOCO models. ChIP-Seq data overview We used GTRD (, release 17 April 2017) as a source of systematically processed ChIP-Seq data. GTRD aggregated ChIP-Seq data from GEO and reprocessed it with a unified pipeline using four different peak calling tools ( macs, gem, pics, sissrs) (–). The peaks were called from the experimental ‘data sets’ (typically a pair of ChIP-Seq experiment and control samples). In some experiments, the control samples were missing, whereas replicated experiments were considered as separate data sets. Four peak sets were obtained from every data set, a peak set for each peak caller. As a starting ground, GTRD provided 3311/2623 data sets and peak sets for 602/354 transcription factors for human/mouse respectively.
The numbers of peaks identified by different peak callers for the same data set were different, which is expected since GTRD used the peak callers with the default parameters and did not normalize for the number of the resulting peaks. However, for the most of the data sets the peak numbers in the peak sets were generally consistent in a sense that all four peak callers agreed in producing a relatively large or a small peak set.
However, for some data sets, there were particular peak callers producing the unexpectedly large number of peaks in discordance with results of other peak callers. We considered such peak sets unreliable and decided not to use them for motif discovery and the subsequent benchmarking. To quantitatively measure the consistency of the numbers of the peaks called by different peak callers we considered the aggregated collection of all data sets for all transcription factors. For each peak caller, an empirical distribution of the peak numbers was constructed from all the peak sets obtained by this peak caller (ignoring the peak sets containing zero peaks).
This allowed us to substitute the number of peaks N in each peak set with the empirical weight of the lighter tail defined as S = min(P (≥ N), P (≤ N)), where P is the empirical probability for a peak set to contain the conditioned number of peaks. Lower values of S correspond to unlikely (extremely large or small) peak numbers.
A concordant data set is expected to have similar values of S for different peak callers. Thus, from 4 peak sets of each data sets, we iteratively excluded the one with the lowest S, until the ratio between the largest and the smallest S in the data set became not 2.
The entire data set was removed if only one peak set remained. This rough filter removed nearly a third of the peak sets. Next, we removed small peak sets of less than 200 peaks since we assumed TFBS models derived from such small data sets as nonrobust. The resulting collection of 8117 / 6189 peak sets for 2885 / 2212 human/mouse ChIP-Seq data sets were used for motif discovery and benchmarking. Shows the number of experimental data sets and peak sets across transcription factors.
Motif discovery The general setup of the motif discovery and analysis was inherited from the HOCOMOCO v10 pipeline. We utilized the top 1000 peaks from each data set: even-ranked peaks were used for motif discovery (training) and odd-ranked peaks as control data for benchmarking.
To rank the peaks based on the ChIP-Seq signal strength we used the following peak caller-specific data: macs – ‘number of tags in the peak region’, gem – ‘immunoprecipitation binding strength’ (the number of immunoprecipitation reads associated with the event), sissrs – ‘NumTags’ (the number of tags supporting the strongest binding site in the reported binding region), pics – ‘enrichment score’ (normalized to the control data). Peaks of sissrs and pics were taken ‘as is’, 300 bp regions around peak summits were taken for macs and gem data. To perform motif discovery and construct classic and dinucleotide position weight matrix models we used ChIPMunk in a new ‘summit’ mode where the base coverage profile of a peak was approximated with a triangle with the vertex at the peak summit location and the base stretched to that of the peak.
This makes the resulting motif occurrences to be correctly ‘phased’ against the peak summit (to reduce the chance of irrelevant patterns being identified). ChIPMunk was set to estimate the background sequence composition automatically (the nucleotide and dinucleotide frequencies for PWMs and dinucleotide PWMs respectively) and executed twice for each peak set: in the default mode and with the single-box motif shape prior. For all peak sets for a particular TF ChIPMunk was set to scan the model length (motif width) range from X down to 7 bp, where X was derived from HOCOMOCO v10 models by incrementing by one the maximum length of known binding models for proteins with similar DNA-binding domain architecture.
To this end for each particular TF we traversed the TFClass hierarchy (,) from TF ‘Subfamily’ up to the ‘Class’ level stopping when binding models from the previous HOCOMOCO version were found. The maximal model length of 23 was used if none of the known models was found. Model curation The motif discovery results were post-processed to ensure that only reliable models entered the benchmarking. First, we used MACRO-APE to compare the newly constructed models with the previously known (HOCOMOCO v10) binding models for the same TF and for the members of the same TF subfamily and family (when such models were available). Next, the results were manually assessed to remove weak patterns (having low information content), patterns inconsistent between different peak sets, patterns belonging to different TFs (e.g. Binding patterns of cofactor proteins or peculiarities of particular experimental data), and to annotate TFs for which binding models were not available or had limited reliability in HOCOMOCO v10. All TFBS models for a particular TF were classified into several categories: the primary models (similar with those previously known for the same TF or consistent within the structural family), the single-box alternative models (resembling the major box of a primary double-box model or the core pattern of the primary model if it was surrounded with long flanking regions), and the alternative models (with a clear alternative pattern reliably discovered from several peak sets).
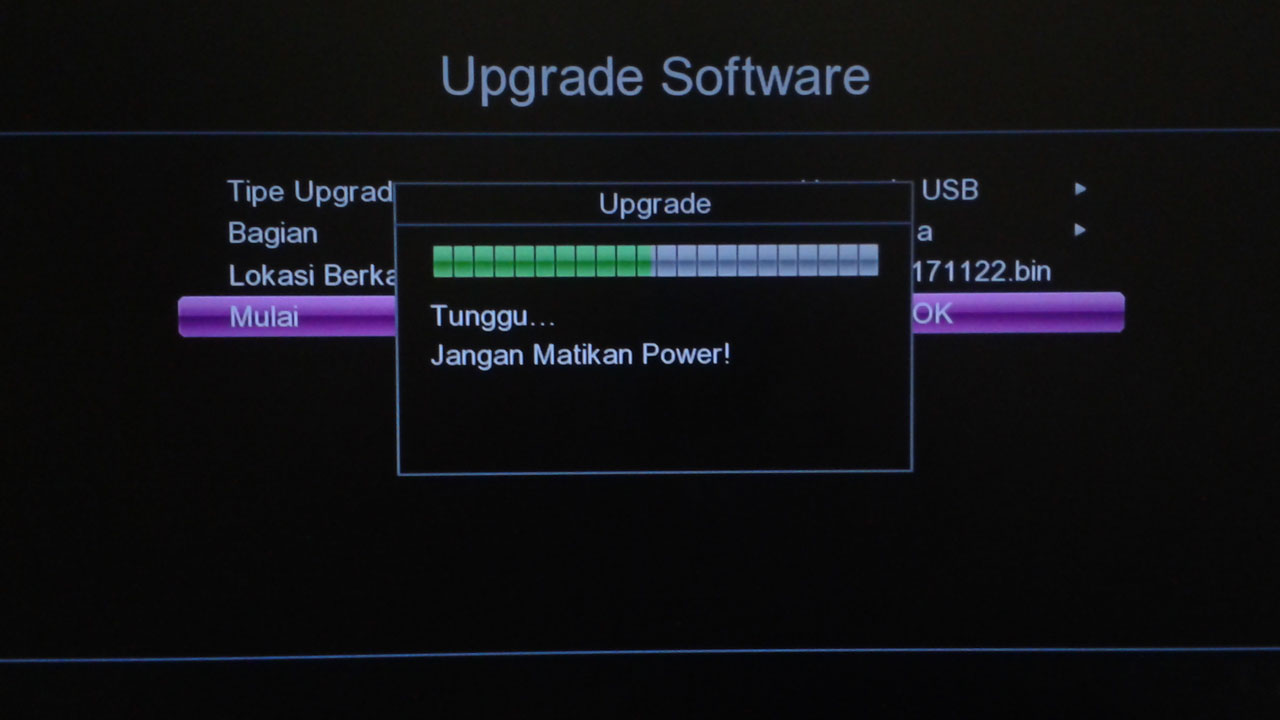
Biss Key Untuk Receiver Skybox
Benchmarking models and assembling the final collection To select the best models for each TF, we used the benchmarking workflow inherited from HOCOMOCO v10 with the following modifications: the ROC (receiver operating characteristic) curves were plotted with the false positive rate axis in the log10 scale. Thus, the AUC logROC was used instead of the AUC ROC (area under curve ROC) in a way similar to that suggested in to prioritize for the ROC region with low false positive rates (see for selected examples of logROC plots). This modification has the same purpose as the partial AUC used in.
A few additional models, e.g. ANDR (androgen receptor) binding model submitted for consideration from , were also considered, yet none of them succeeded in the benchmark except for the models that we specifically designed to describe binding sites subtypes, e.g. Genuine binding sites of NANOG transcription factor (see Discussion). Some TFs were notably better represented by the experimental data from either human or mouse orthologs. To benefit from all available data, we tested TF binding models from both species (human and mouse) on both human and mouse data.
This allowed us to evaluate the quality of ‘human’ binding models taking into account mouse data and vice versa. We used the following strategy to include cross-species AUC logROC ( ) values in the final quality estimation.
For each species (human or mouse) and for each model we computed weighted AUC logROC value. This allowed us to take into account all available data for all TFs, which was especially important for the cases when only a limited number of experimental data sets was available. The resulting values of were used to select the best primary model for each TF and to rank alternative models. Sometimes the model based on the mouse data was assigned to a human TFs as performing systematically better at human data in the benchmark. The same was true for some human models outperforming all mouse models at the corresponding mouse data (see Results).
In the previous releases, the HOCOMOCO models were provided with simplified A-to-D quality ratings used to distinguish models that properly characterize the TF binding specificity (A to C quality) from models of limited predictive power and those based on limited experimental evidence (D quality). We adopt the same scheme in HOCOMOCO v11: the models of A, B, and C quality are recommended to make reliable de novo predictions, whereas models of D quality are still useful for various exploratory purposes, e.g., family-wide TF analysis. The final model quality assignment procedure was inspired by HOCOMOCO v10 but modified to benefit from the data of multiple peak callers.
For the quality assignment, as in HOCOMOCO v10, we used linear, rather than logarithmic, AUC ROC scores and AUC thresholds (minimal = 0.65, optimal = 0.8) to maintain consistency with the original HOCOMOCO v10 quality ratings. At the benchmark stage, we performed a comprehensive cross-species evaluation of the models. For 118 out of 384 benchmarked TFs, the best TFBS model for human data was the one derived from a mouse peak set. Similarly, for 85 out of 322 TFs the best model for mouse data was a model derived from a human peak set.
This means that for a particular TF differences in binding patterns between species are less obvious than between experimental conditions or cell types. This observation allowed us to make a cross-species transfer of models between human and mouse orthologous TFs. For some TFs, the ChIP-Seq data were available only for one of the two species. In such cases, we conducted a cross-species transfer of the models if the respective TF was previously included in the HOCOMOCO v10 set for the target species. The respective quality of the new cross-species model was set one category lower (e.g., A substituted by B) to account for the uncertainty introduced by a cross-species transfer. If the resulting quality has been better or equal to that of the existing HOCOMOCO v10 model, the new model from a cross-species transfer has been adopted for v11.
Otherwise, the existing v10 model has been kept. The quality values directly inherited (non-benchmarked) HOCOMOCO v10 S-models (describing secondary patterns) have been set one category lower than those of the primary models. At this step, we re-examined D-quality models of HOCOMOCO v10 and excluded those that were not confirmed by the ChIP-Seq data such as YBX1. By doing so, unreliable binding models for eight human and three mouse TFs previously included in HOCOMOCO v10 were discarded. The HOCOMOCO v11 model identifiers have the following format: ‘X.H11MO.Y.Z’ where X is the UniProt ID for the TF, Y is the model rank, and Z is the model quality rating.
Dinucleotide models are labeled with ‘H11DI’. If the curation stage yielded several models (primary, single-box, etc.), they were ranked based on AUC logROC with the best model being ranked as 0 and 1–2 ranks assigned to alternative models. RESULTS As compared with the previous release (v10), in HOCOMOCO v11 we increased the number of mononucleotide models by 131/105 (for human/mouse respectively) and thus additionally covered 88/61 TFs (totaling to 771/531 models and 680/453 covered TFs). The overall performance of the models was notably improved (Figure ), in particular, 31 human models from HT-SELEX data were replaced by the new ChIP-Seq-based models. In regards to the structural classification of transcription factors , the class of C2H2 zinc finger factors profited the most from the update growing from 92 to 159 binding models. Number of TFs (Y-axis) with the best available model of a given quality (X-axis). The number of the most reliable A-quality models is more than doubled in HOCOMOCO v11 comparing to v10.
The collection of dinucleotide PWMs that covers only primary models has also been notably expanded with 227 TFs (totaling to 313) for human and 211 TFs (totaling to 263 TFs) for mouse. An interesting by-product of our analysis was the comparison of the peak callers regarding the quality of the TFBS models derived from the respective peak sets. To this end, we used the motif quality metrics already obtained at the benchmarking step and estimated the number of transcription factors for which the best available model was derived from the peak set produced by a particular peak caller.
To rank the peaks produced by each peak caller we selected the measure that was the most similar to a peak height, and performed motif discovery and validation based on the highest peaks. The resulting TFBS models had notably different performance. The best peak caller was gem, as it was the source of the best TFBS models across the widest range of TFs. The popular macs appeared close second. The remaining two peak callers ( sissrs and pics) performed noticeably poorer. This might be one of the reasons for the quality improvement of HOCOMOCO v11, because v10 was based solely on sissrs. Figure shows the total number of human and mouse TFs for which the best models were derived from peaks of the particular peak caller.
Dongle Wifi Untuk Receiver
DISCUSSION For many TFs the general HOCOMOCO pipeline was successful in constructing and benchmarking the TFBS models. However, multiple questions remain open. In particular, for many TFs (including many homeodomain and putatively AT-rich binding TFs) we failed to construct reliable binding models. Some cases might be a consequence of the limited data quality, but there were dozens of TFs with multiple ChIP-Seq data sets for which our procedure failed to discover a clear pattern consistent across data sets.
One of such cases is ARID3A, which is expected to bind AT-rich patterns according to previously available in vitro data. However, we failed to identify any common binding pattern in vivo, and also, no common pattern could be found in FactorBook data as well. Thus, a general question remains: which fraction of TFs do not exhibit clear binding patterns in vivo and if this is the case, is this determined by functional non-specific binding related to the architecture of the DNA-binding domains? The alternative explanation might be the prevalent indirect DNA binding or just a limited quality of the currently available antibodies. Models of SMAD TF family make up another example.
It appears that there are fuzzy but still visible patterns in aligned TFBS for these TFs. It is not clear whether these TFs require alignment-free TFBS models or if they correspond to alignable but multiple motif subtypes (Figure ). Examples of TFBS models included in HOCOMOCO v11: ( A) SMAD2/3 models exhibit fuzzy patterns; ( B) two variants of ANDR composite elements: the palindromic site and the double box ANDR-FOX; ( C) the ‘genuine’ NANOG binding site along the putative OCT4-SOX2/NANOG composite element (for clarity the nucleotide pileups are shown unscaled); ( D) SP1/2 (canonical SP-family GC-box) and SP7 binding models. Other non-trivial cases include composite elements detected as primary models. One surprising example is ANDR-FOX composite element detected as a primary model for ANDR (Figure ). Another non-trivial case is NANOG with the respective ChIP-Seq data stably yielding SOX2-OCT4 composite element as a primary model. Though it has been proposed that OCT4 (POU5F1), SOX2 and NANOG can potentially form a composite element , further analysis is needed to prove this hypothesis.
The ‘genuine’ homeodomain-like NANOG pattern can be found in mouse ChIP-Seq data with differential motif discovery by contrasting NANOG ChIP-Seq with that of OCT4. It is possible to obtain similar results using ChIPMunk by excluding the regions overlapping OCT4 and SOX2 peaks from the training NANOG sequence set (Figure ). The resulting ‘genuine’ NANOG model is in better agreement with model from SELEX experiments and shows good performance reaching B/A rating on human/mouse ChIP-Seq data. On a broader scale, one can observe, in general, a good similarity between TF binding models for TFs belonging to the same structural families. However, there are especially interesting cases such as GC-box binding Sp-family of TFs. The TFs with newly processed ChIP-Seq data include SP7, which binds a completely different pattern (Figure ). The model was rejected during automatic annotation but restored at the manual curation stage based on evidence from.
One of the distinguishing features of HOCOMOCO is the unified pipeline of motif discovery and benchmarking. For HOCOMOCO v11 we performed a careful reprocessing of the experimental data rather than the aggregation of TFBS models reported by other studies (–).

Our approach has its disadvantages. Specifically, the compulsory curation of the discovered models is dependent on external data, e.g. UniProt , TF Encyclopedia , and the JASPAR database (,), which we consulted at the model curation stage. Practical usage of HOCOMOCO models Different practical applications of HOCOMOCO require different subsets of models. In the case of TFBS prediction for the analysis of regulatory networks, the D-quality models may notably reduce performance. However, other applications such as annotation of regulatory sequence variants (,) may benefit from additional TFs and alternative binding models.
Thus, starting from this release we provide two collections: the CORE collection of the most precise models (ABC quality) recommended for TFBS predictions for annotation of regulatory regions, and the FULL collection that includes alternative models and models of lower quality (D), which can be used for exploratory purposes. By design, the dinucleotide collection is aimed specifically for precise TFBS prediction and includes only primary ABC quality models. To facilitate practical applications, we continue to provide P-value-to-score-threshold mappings for all models and the complete collection dump in various formats (including plain text, TRANSFAC and MEME).
As in HOCOMOCO v9 and v10, we continue to provide a subset of default thresholds at fixed motif P-values with the default recommended P-value of 0.0005 (a single expected prediction per 1000 bp of a two-stranded random sequence). At this level HOCOMOCO v11 model reaches median sensitivity of 0.75, i.e. Typically recognizes three of four binding sites in the positive control data. When it comes to motif finding, HOCOMOCO now has several options including a command-line tool (SPRY-SARUS) and two web-interfaces: MoLoTool for visual inspection of shorter sequences, and the HOCOMOCO-in-BioUML to scan extensive genomic regions.
Marking motif occurrences with MoLoTool Major motif analysis software (,) include methods for motif finding, i.e., to detect occurrences of given motifs in a user-defined set of sequences of genomic regions. Practical applications often require detailed analysis of particular DNA sequences in the vicinity of TFBS at a single-nucleotide resolution, e.g. To rationally change TFBS affinity by site-specific mutations. To facilitate such local analysis with HOCOMOCO v11 models, we designed MoLoTool (Motif Location Toolbox) which allows scanning a given set of sequences for occurrences of user-selected PWMs. The basic workflow is very simple: (a) select a desired subset of TFBS models from an interactive catalog, (b) paste or upload a set of DNA sequences and (c) submit the form to obtain not only the table view of predicted TFBS but also the colored sequence-based map of motif occurrences. Lexicon pcm native reverb plug in bundle vst rtas v1.1.3-air. The main advantage of MoLoTool is its ability to produce text-based colored markings which can be directly copied-and-pasted into any rich text editor (e.g., MS Word). The TFBS predictions depend on the motif P-value threshold that can be dynamically adjusted to seamlessly update the markings without reloading the web page.
An example of the MoLoTool markup is shown in. CONCLUSIONS To summarize, we report a major update of the HOCOMOCO collection (v11) that contains binding models of the increased quality for 453 mouse and 680 human transcription factors and includes 1302 mononucleotide and 576 dinucleotide PWMs.
The update was constructed by systematic motif discovery and benchmarking based on more than five thousand ChIP-Seq experiments processed with four ChIP-Seq peak calling tools. We complement HOCOMOCO by MoLoTool that can perform visual mapping of HOCOMOCO binding sites in DNA sequences. We believe that the current version of HOCOMOCO can be used for diverse applications in fundamental and applied research from global TFBS prediction to the annotation of regulatory sequence variants.